Researchers from Facebook, it seems, has found a way to use machine learning in order to give applications the Oculus Quest is 67% more opportunities to work with GPUs.
Oculus Quest is a standalone headset, which means that the computing hardware is inside the device. Due to limitations in size and capacity, and desires to sell the device at a relatively affordable price, the Quest uses a chip of a smartphone is much less powerful than a gaming PC.
“The new generation VR and AR will require finding new, more effective ways of rendering high quality graphics with low latency” (Facebook)
The new technique works by rendering at a lower resolution than usual, then the vision center scales using the algorithm of “superresolution” of machine learning. These algorithms have become popular in the last few years, with some sites even allow users to upload any image to your PC or phone to increase quality.
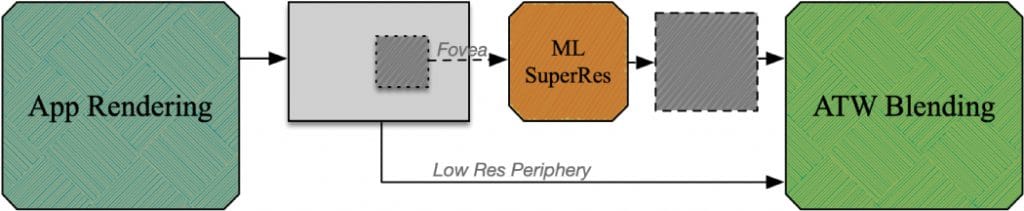
When sufficient training data, the algorithms of superresolution can produce much more detailed output than traditional upscaling (increase image resolution). Although just a few years ago “Zoom and Enhance” was a meme used to ridicule by those who mistakenly believed that the computers can do it, machine learning has made this idea a reality.
One of the authors is Bastani Behnam, head of the Department of graphic Facebook in the Core AR/VR Technologies. In the period from 2013 to 2017 Bastani worked at Google, developing “advanced display system”, and then led the development of the rendering pipeline Daydream.
It is interesting to note that in fact we are not talking about the algorithm of superresolution or liberation of GPU with it. A direct goal of researchers was to create a “Foundation” for running machine learning algorithms in real-time in the current rendering pipeline (with low latency), whom they reached. Increasing the resolution to superresolution in fact is only the first example that it works.
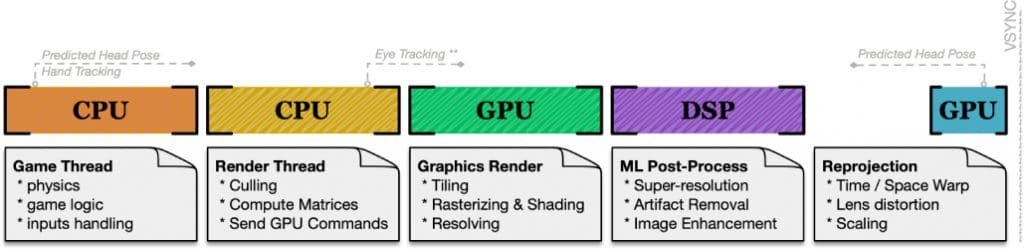
The researchers argue that when rendering with 70% lower resolution in each direction method can save about 40% of the time GPU, and the developers can “use these resources to create the best content.”
In applications such as “media viewer”, the saved power of the GPU can be left unused for longer battery life, because the Snapdragon chips (and most other) DSP (used for such machine learning tasks like this one) is much more energy efficient than the GPU.
Apparently, the use of superresolution is to save power of the GPU is only one potential application of this infrastructure in the rendering pipeline:
“In addition to the application of super-resolution, the platform can also be used in the removal of compression artifacts for streaming content, forecasting human resources, analyzing the characteristics and feedback for managed rendering. We believe that the use of computational methods and machine learning in the mobile graphics pipeline will provide us with many opportunities for mobile graphics for the next generation” (Facebook)
Source